Model Fitness and Predictive Accuracy in Linear Mixed-Effects Models with Latent Clusters
Keywords:
Clustered data, Primal and dual clusters, Linear mixed-effects models, Model fitness, Predictive accuracyAbstract
In clustered data, observations within a cluster show similarity between themselves because they share common features different from observations in the other clusters. In a given population, different clustering may surface because correlation may occur across more than one dimension. The existing multilevel analysis techniques of the primal linear mixed-effect models are limited to natural clusters which are often not realistic to capture in real-life situations. Therefore, this paper proposes dual linear mixed models (DLMMs) for modeling unobserved latent clusters when such are present in data sets to yield appreciable gains in model fitness and predictive accuracy. The methodology explored the development and analysis of the dual linear mixed models (DLMMs) based on the derived latent clusters from the natural clusters using multivariate cluster analysis. A published data set on political analysis was used to demonstrate the efficiency of the proposed models. The proposed DLMMs have yielded minimum values of the models' assessment criteria (Akaike information criterion, Bayesian information criterion, and root mean squared error), and hence, outperformed the classical PLMMs in terms of model fitness and predictive accuracy.
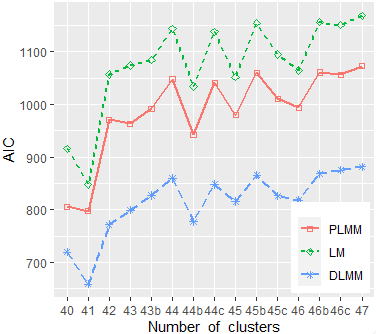
Published
How to Cite
Issue
Section
Copyright (c) 2023 Yusuf Bello, Waheed Yahya, B., Professor

This work is licensed under a Creative Commons Attribution 4.0 International License.