On the fourth-order hybrid beta polynomial kernels in kernel density estimation
Keywords:
Kernel density estimation, Fourth-order kernels, hybrid kernels, AMISE, EfficiencyAbstract
This paper introduces a novel family of fourth-order hybrid beta polynomial kernels tailored for statistical analysis. The efficacy of these kernels is evaluated using two principal performance metrics: asymptotic mean integrated squared error (AMISE) and kernel efficiency. Comprehensive assessments were conducted using both simulated and real-world datasets, enabling a thorough comparison with conventional fourth-order polynomial kernels. The evaluation process entailed computing AMISE and efficiency metrics for both the hybrid and classical kernels. Consistently, the results illustrated the superior performance of the hybrid kernels over their classical counterparts across diverse datasets, underscoring the robustness and effectiveness of the hybrid approach. By leveraging these performance metrics and conducting evaluations on simulated and real world data, this study furnishes compelling evidence supporting the superiority of the proposed hybrid beta polynomial kernels. The heightened performance, evidenced by lower AMISE values and elevated efficiency scores, strongly advocates for the adoption of the proposed kernels in statistical analysis tasks, presenting a marked improvement over traditional kernels.
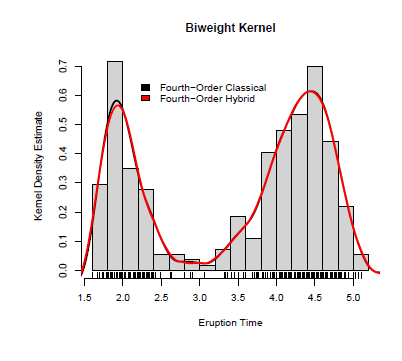
Published
How to Cite
Issue
Section
Copyright (c) 2023 Benson Ade Eniola Afere

This work is licensed under a Creative Commons Attribution 4.0 International License.